Mohammed Abuhamad
I am an assistant professor of Computer Science at Loyola University Chicago . I received a Ph.D. degree in Computer Science from the University of Central Florida (UCF) in 2020. I also received a Ph.D. degree in Electrical and Computer Engineering from INHA University , (Incheon, Republic of Korea) in 2020. I received a Master degree in Information Technology (Artificial Intelligence) from the National University of Malaysia , (Bangi, Malaysia) in 2013.
I am interested in the fields of AI/Deep-Learning Security and Software and Mobile/IoT Security, with a focus on ensuring the robustness and privacy of AI models in adversarial settings. I work on enhancing machine learning applications in security-critical systems, such as autonomous vehicles, robotics, and IoT devices, while ensuring privacy and efficiency. I have published several peer-reviewed research papers in top-tier conferences and journals such as ACM CCS, PoPETS, IEEE TDSC, IEEE TIFS, IEEE IoT-J, and IEEE ICDCS.
Research Lab:
Cybersecurity lab and AI for Secure Computing Research Lab ( AISeC) .
Teaching (Fall 2024):
COMP 358/458: Big Data Analytics |
COMP 388/487: Deep Learning.
Office Hours: Monday 03:00 - 05:00 PM | Friday 09:00 - 11:00 AM
Information Security
We employ advances in machine learning to information and system security.
Software Security
We study methods for software authorship and vulnerabilities.
Privacy
Authorship anonymization and behavioral biometrics for authentication.
Robustness and Adversarial ML
We analyze the security proprieties of machine learning models
Applied Machine Learning
We study and employ machine learning in various domains.
Malware Analysis
We utilize various characterizing features to build machine learning models to detect and analyze malware.
Research
To improve our understanding of systems, and to guide security analytics towards secure systems design, I employ advances in deep machine learning to systems security. The emergence of such learning techniques promises various avenues for attribution in data-driven security analytics, and those techniques constitute the cornerstone of my current and future research interests. My current research contributions have been focused on creating efficient and accurate methods for software security, building, customizing, optimizing, and leveraging deep machine learning techniques for security and privacy.
Information Security
Machine Learning
Software and IoT Security
Data Intelligence
Research Interests
We work on developing methods and algorithms to understand information from different sources and with different formats.
Information Security and Privacy
Secure Computing
AI for social good
Publications
Research Projects
Robustness and Security of AI
Deep Neural Networks have achieved state-of-the-art performance in various applications. It is crucial to verify that the high accuracy prediction for a given task is derived from the correct problem representation and not from the misuse of artifacts in the data.
Investigating the security proprieties of machine learning models, recent studies have shown various categories of adversarial attacks such as model leakage, data membership inference,
model confidence reduction, evasion, and poisoning attacks.
We believe it is important to investigate and understand the implications of such attacks on sensitive applications in the field of information security and privacy.
Malware Analysis and Software Security
Malware is one of the serious computer security threats. To protect computers from infection, accurate detection of malware is essential. At the same time, malware detection faces two main practical challenges: the speed of malware development and their distribution continues to increase with complex methods to evade detection (such as a metamorphic or polymorphic malware). This project utilizes various characterizing features extracted from each malware using static and dynamic analysis to build seven machine learning models to detect and analyze malware. We investigate the robustness of such machine learning models against adversarial attacks.
Network Security and Online Privacy
Network monitoring applications such as flow analysis, intrusion detection, and performance monitoring have become increasingly popular owing to the continuous increase in the speed and volume of network traffic. We work on investigating the feasibility of an in-network intrusion detection system that leverages the computation capabilities of commodity switches to facilitate fast and real-time response. Moreover, we explore the traffic sampling techniques that preserve flows’ behavior to apply intelligence in network monitoring and management. We also address the increased privacy concerns regarding website fingerprinting attacks despite the popular anonymity tools such as Tor and VPNs.
Sponsors
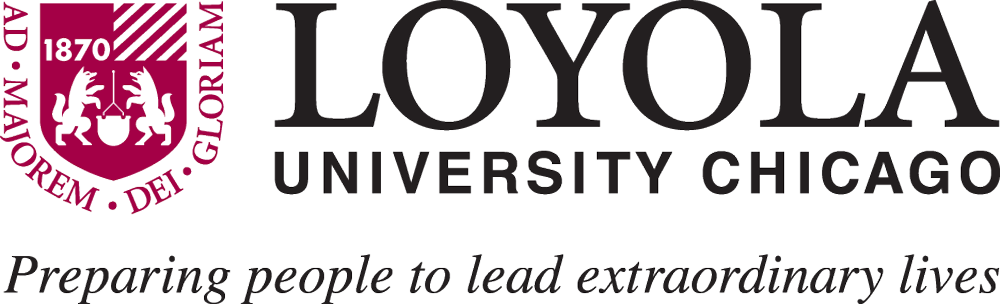
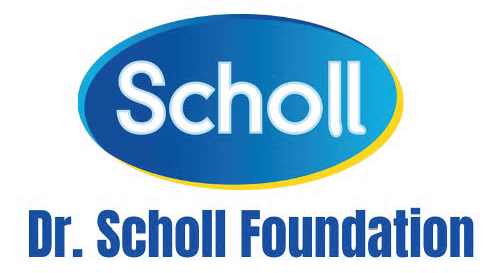
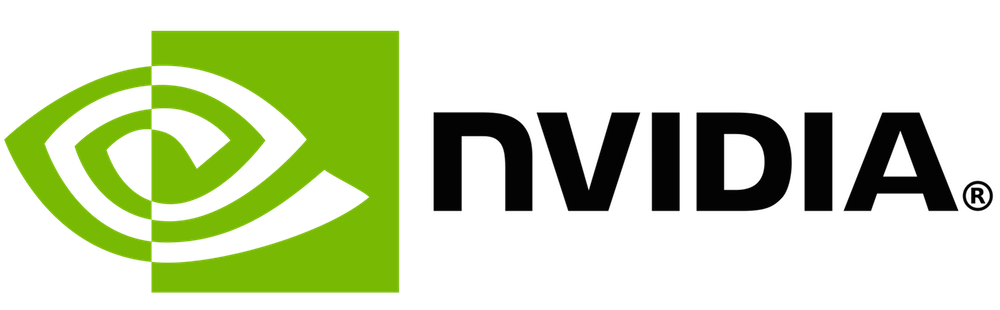
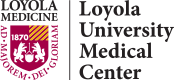
Contact Us
Our Address
213 Doyle Center, Lake Shore Campus
1052 W Loyola Ave. Chicago, IL 60626
Email Us
Call Us
+1 773 508 3557